\(\ell_1\) 趋势滤波
Judon Wilson,在2014年5月28日的基础上进行了衍生改编。改编自Kwangmoo Koh在2007年12月10日的同名CVX示例
主题参考:
S.-J. Kim, K. Koh, S. Boyd, and D. Gorinevsky,``l_1 Trend Filtering’’、 http://stanford.edu/~boyd/papers/l1_trend_filter.html
介绍
在许多领域中,需要估计时间序列数据中的潜在趋势。l_1
趋势滤波方法可以产生从时间序列``y`` 中得到的分段线性的趋势估计值``x``。
l_1
趋势估计问题可以表述为:
\[\begin{array}{ll}
\mbox{最小化} & (1/2)||y-x||_2^2 + \lambda ||Dx||_1,
\end{array}\]
其中,变量为 \(x\) ,问题数据为 \(y\) 和 \(\lambda\) ,满足 \(\lambda >0\)。:math:`D`为二阶差分矩阵,行数是….. math:: begin{bmatrix}0 & cdots & 0 & -1 & 2 & -1 & 0 & cdots & 0 end{bmatrix}.
CVXPY 不适用于 \(\ell_1\) 趋势过滤问题。 对于大规模问题,请使用 l1_tf (https://www.stanford.edu/~boyd/l1_tf/)。
制定和解决问题
import numpy as np
import cvxpy as cp
import scipy as scipy
import cvxopt as cvxopt
# 加载时间序列数据:S&P 500 价格的对数。
y = np.loadtxt(open('data/snp500.txt', 'rb'), delimiter=",", skiprows=1)
n = y.size
# 形成二阶差分矩阵。
e = np.ones((1, n))
D = scipy.sparse.spdiags(np.vstack((e, -2*e, e)), range(3), n-2, n)
# 设置正则化参数。
vlambda = 50
# 解决 l1 趋势过滤问题。
x = cp.Variable(shape=n)
obj = cp.Minimize(0.5 * cp.sum_squares(y - x)
+ vlambda * cp.norm(D*x, 1) )
prob = cp.Problem(obj)
# ECOS 和 SCS 求解器在迭代次数限制之前无法收敛。改用 CVXOPT。
prob.solve(solver=cp.CVXOPT, verbose=True)
print('求解器状态: {}'.format(prob.status))
# 检查错误。
if prob.status != cp.OPTIMAL:
raise Exception("求解器未收敛!")
print("最优目标值: {}".format(obj.value))
pcost dcost gap pres dres k/t
0: 0.0000e+00 -1.0000e+00 1e+05 1e-01 4e-02 1e+00
1: 2.2350e-01 1.5374e-01 8e+03 8e-03 3e-03 7e-02
2: 1.9086e-01 2.3346e-01 1e+03 1e-03 4e-04 6e-02
3: 3.9403e-01 4.4110e-01 7e+02 7e-04 3e-04 6e-02
4: 3.5979e-01 4.1278e-01 3e+02 3e-04 1e-04 6e-02
5: 6.4154e-01 6.4522e-01 2e+01 2e-05 7e-06 4e-03
6: 9.0480e-01 9.0710e-01 1e+01 1e-05 4e-06 3e-03
7: 9.9603e-01 9.9825e-01 1e+01 1e-05 4e-06 2e-03
8: 1.0529e+00 1.0542e+00 6e+00 6e-06 2e-06 1e-03
9: 1.1994e+00 1.2004e+00 4e+00 4e-06 2e-06 1e-03
10: 1.2689e+00 1.2693e+00 2e+00 2e-06 6e-07 4e-04
11: 1.3728e+00 1.3729e+00 5e-01 5e-07 2e-07 1e-04
12: 1.3802e+00 1.3803e+00 2e-01 2e-07 9e-08 6e-05
13: 1.3965e+00 1.3965e+00 1e-01 1e-07 4e-08 3e-05
14: 1.3998e+00 1.3998e+00 3e-02 3e-08 1e-08 8e-06
15: 1.3999e+00 1.3999e+00 3e-02 3e-08 1e-08 7e-06
16: 1.4011e+00 1.4011e+00 9e-03 9e-09 3e-09 2e-06
17: 1.4013e+00 1.4013e+00 3e-03 3e-09 1e-09 8e-07
18: 1.4014e+00 1.4014e+00 6e-04 6e-10 3e-10 2e-07
19: 1.4014e+00 1.4014e+00 2e-04 2e-10 7e-11 4e-08
20: 1.4014e+00 1.4017e+00 4e-05 4e-11 2e-08 1e-08
21: 1.4014e+00 1.4015e+00 3e-06 4e-12 8e-08 7e-10
22: 1.4014e+00 1.4013e+00 4e-08 4e-13 2e-08 9e-12
找到最优解。
求解器状态: 最优
最优目标值: 1.4014300716775199
结果图
import matplotlib.pyplot as plt
# 在 ipython 中内联显示图形。
%matplotlib inline
# 图形属性。
plt.rc('text', usetex=True)
plt.rc('font', family='serif')
font = {'weight' : 'normal',
'size' : 16}
plt.rc('font', **font)
# 绘制原始信号的估计趋势。
plt.figure(figsize=(6, 6))
plt.plot(np.arange(1,n+1), y, 'k:', linewidth=1.0)
plt.plot(np.arange(1,n+1), np.array(x.value), 'b-', linewidth=2.0)
plt.xlabel('日期')
plt.ylabel('对数价格').. parsed-literal::
Text(0, 0.5, 'log price')
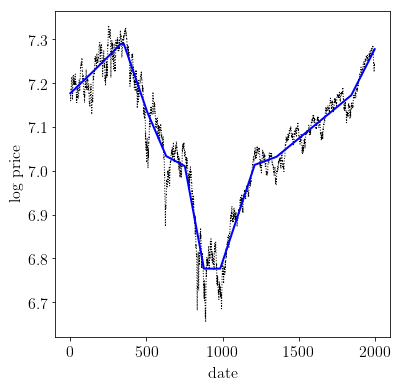