分析: 评估和结果分析
介绍
分析
旨在显示 日内交易
的图形报告,帮助用户以可视化方式评估和分析投资组合。以下是一些可以查看的图形:
- 位置分析
报告图
信息系数图
累积收益图
风险分析图
排名标签图
- 模型分析
模型表现图
在 Qlib 中,所有累积利润指标(例如回报率、最大回撤)都是通过求和计算的。 这避免了指标或图表随着时间的推移呈指数级增加。
图形报告
用户可以运行以下代码获取所有支持的报告。
>> import qlib.contrib.report as qcr
>> print(qcr.GRAPH_NAME_LIST)
['analysis_position.report_graph', 'analysis_position.score_ic_graph', 'analysis_position.cumulative_return_graph', 'analysis_position.risk_analysis_graph', 'analysis_position.rank_label_graph', 'analysis_model.model_performance_graph']
备注
如需详细信息,请参考函数文档:类似于``help(qcr.analysis_position.report_graph)``
用法和示例
使用 analysis_position.report 的用法
API
- qlib.contrib.report.analysis_position.report.report_graph(report_df: DataFrame, show_notebook: bool = True) [<class 'list'>, <class 'tuple'>]
display backtest report
Example:
import qlib import pandas as pd from qlib.utils.time import Freq from qlib.utils import flatten_dict from qlib.backtest import backtest, executor from qlib.contrib.evaluate import risk_analysis from qlib.contrib.strategy import TopkDropoutStrategy # init qlib qlib.init(provider_uri=<qlib data dir>) CSI300_BENCH = "SH000300" FREQ = "day" STRATEGY_CONFIG = { "topk": 50, "n_drop": 5, # pred_score, pd.Series "signal": pred_score, } EXECUTOR_CONFIG = { "time_per_step": "day", "generate_portfolio_metrics": True, } backtest_config = { "start_time": "2017-01-01", "end_time": "2020-08-01", "account": 100000000, "benchmark": CSI300_BENCH, "exchange_kwargs": { "freq": FREQ, "limit_threshold": 0.095, "deal_price": "close", "open_cost": 0.0005, "close_cost": 0.0015, "min_cost": 5, }, } # strategy object strategy_obj = TopkDropoutStrategy(**STRATEGY_CONFIG) # executor object executor_obj = executor.SimulatorExecutor(**EXECUTOR_CONFIG) # backtest portfolio_metric_dict, indicator_dict = backtest(executor=executor_obj, strategy=strategy_obj, **backtest_config) analysis_freq = "{0}{1}".format(*Freq.parse(FREQ)) # backtest info report_normal_df, positions_normal = portfolio_metric_dict.get(analysis_freq) qcr.analysis_position.report_graph(report_normal_df)
- 参数:
report_df –
df.index.name must be date, df.columns must contain return, turnover, cost, bench.
return cost bench turnover date 2017-01-04 0.003421 0.000864 0.011693 0.576325 2017-01-05 0.000508 0.000447 0.000721 0.227882 2017-01-06 -0.003321 0.000212 -0.004322 0.102765 2017-01-09 0.006753 0.000212 0.006874 0.105864 2017-01-10 -0.000416 0.000440 -0.003350 0.208396
show_notebook – whether to display graphics in notebook, the default is True.
- 返回:
if show_notebook is True, display in notebook; else return plotly.graph_objs.Figure list.
图形结果
备注
X 轴:交易日
- Y 轴:
- cum bench
基准累计收益系列
- cum return wo cost
无成本的投资组合累计收益系列
- cum return w cost
有成本的投资组合累计收益系列
- return wo mdd
无成本累计收益的最大回撤系列
- return w cost mdd:
有成本累计收益的最大回撤系列
- cum ex return wo cost
投资组合与无成本基准比较的累计异常收益(CAR)系列
- cum ex return w cost
投资组合与有成本基准比较的累计异常收益(CAR)系列
- turnover
换手率系列
- cum ex return wo cost mdd
无成本累计异常收益(CAR)的回撤系列
- cum ex return w cost mdd
有成本累计异常收益(CAR)的回撤系列
上面的阴影部分:对应于 cum return wo cost 的最大回撤
下面的阴影部分:对应于 cum ex return wo cost 的最大回撤
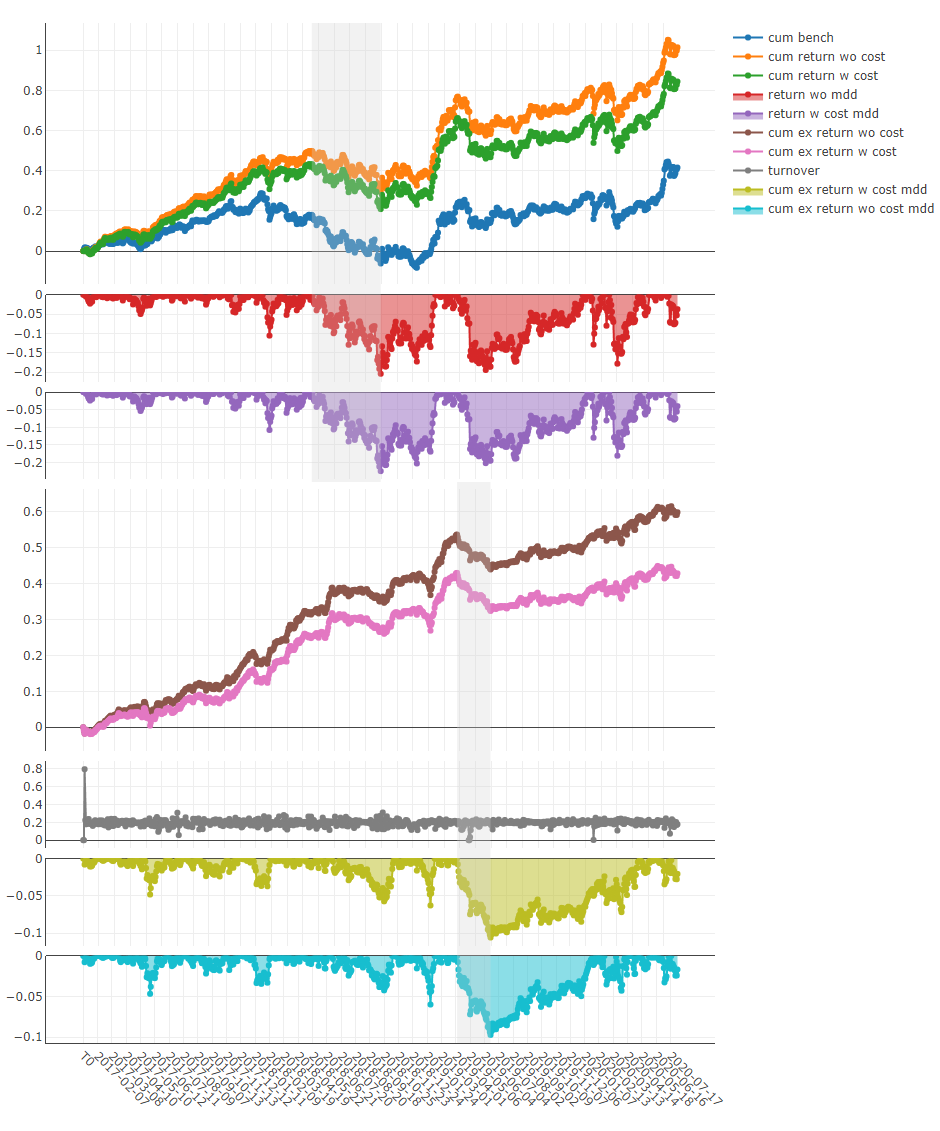
使用 analysis_position.score_ic
API
- qlib.contrib.report.analysis_position.score_ic.score_ic_graph(pred_label: DataFrame, show_notebook: bool = True, **kwargs) [<class 'list'>, <class 'tuple'>]
score IC
Example:
from qlib.data import D from qlib.contrib.report import analysis_position pred_df_dates = pred_df.index.get_level_values(level='datetime') features_df = D.features(D.instruments('csi500'), ['Ref($close, -2)/Ref($close, -1)-1'], pred_df_dates.min(), pred_df_dates.max()) features_df.columns = ['label'] pred_label = pd.concat([features_df, pred], axis=1, sort=True).reindex(features_df.index) analysis_position.score_ic_graph(pred_label)
- 参数:
pred_label –
index is pd.MultiIndex, index name is [instrument, datetime]; columns names is [score, label].
instrument datetime score label SH600004 2017-12-11 -0.013502 -0.013502 2017-12-12 -0.072367 -0.072367 2017-12-13 -0.068605 -0.068605 2017-12-14 0.012440 0.012440 2017-12-15 -0.102778 -0.102778
show_notebook – whether to display graphics in notebook, the default is True.
- 返回:
if show_notebook is True, display in notebook; else return plotly.graph_objs.Figure list.
图形结果
备注
X 轴:交易日
- Y 轴:
- ic
label 与 prediction score 之间的 Pearson 相关系数 系列。 在上面的示例中,label 被定义为 Ref($close, -2)/Ref($close, -1)-1。请参考 数据特征 了解更多细节。
- rank_ic
label 与 prediction score 之间的 Spearman 秩相关系数 系列。
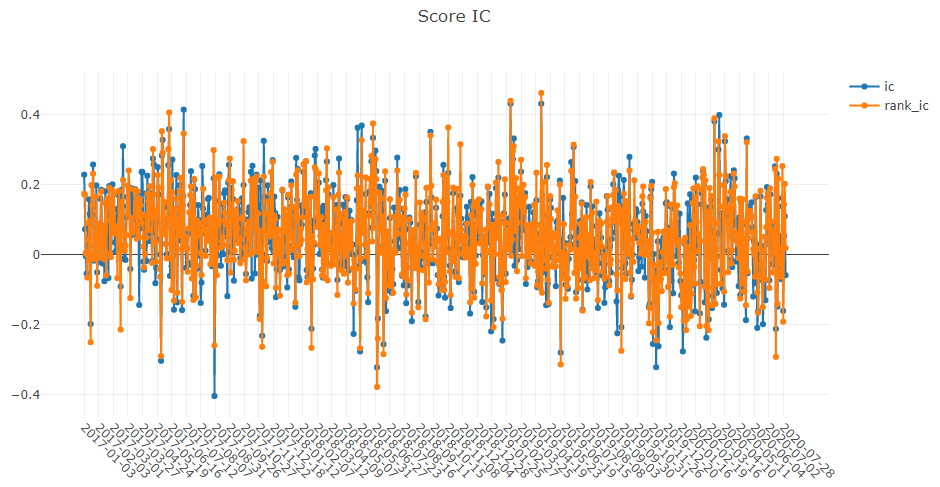
使用 analysis_position.risk_analysis 的方法
API
- qlib.contrib.report.analysis_position.risk_analysis.risk_analysis_graph(analysis_df: DataFrame | None = None, report_normal_df: DataFrame | None = None, report_long_short_df: DataFrame | None = None, show_notebook: bool = True) Iterable[Figure]
Generate analysis graph and monthly analysis
Example:
import qlib import pandas as pd from qlib.utils.time import Freq from qlib.utils import flatten_dict from qlib.backtest import backtest, executor from qlib.contrib.evaluate import risk_analysis from qlib.contrib.strategy import TopkDropoutStrategy # init qlib qlib.init(provider_uri=<qlib data dir>) CSI300_BENCH = "SH000300" FREQ = "day" STRATEGY_CONFIG = { "topk": 50, "n_drop": 5, # pred_score, pd.Series "signal": pred_score, } EXECUTOR_CONFIG = { "time_per_step": "day", "generate_portfolio_metrics": True, } backtest_config = { "start_time": "2017-01-01", "end_time": "2020-08-01", "account": 100000000, "benchmark": CSI300_BENCH, "exchange_kwargs": { "freq": FREQ, "limit_threshold": 0.095, "deal_price": "close", "open_cost": 0.0005, "close_cost": 0.0015, "min_cost": 5, }, } # strategy object strategy_obj = TopkDropoutStrategy(**STRATEGY_CONFIG) # executor object executor_obj = executor.SimulatorExecutor(**EXECUTOR_CONFIG) # backtest portfolio_metric_dict, indicator_dict = backtest(executor=executor_obj, strategy=strategy_obj, **backtest_config) analysis_freq = "{0}{1}".format(*Freq.parse(FREQ)) # backtest info report_normal_df, positions_normal = portfolio_metric_dict.get(analysis_freq) analysis = dict() analysis["excess_return_without_cost"] = risk_analysis( report_normal_df["return"] - report_normal_df["bench"], freq=analysis_freq ) analysis["excess_return_with_cost"] = risk_analysis( report_normal_df["return"] - report_normal_df["bench"] - report_normal_df["cost"], freq=analysis_freq ) analysis_df = pd.concat(analysis) # type: pd.DataFrame analysis_position.risk_analysis_graph(analysis_df, report_normal_df)
- 参数:
analysis_df –
analysis data, index is pd.MultiIndex; columns names is [risk].
risk excess_return_without_cost mean 0.000692 std 0.005374 annualized_return 0.174495 information_ratio 2.045576 max_drawdown -0.079103 excess_return_with_cost mean 0.000499 std 0.005372 annualized_return 0.125625 information_ratio 1.473152 max_drawdown -0.088263
report_normal_df –
df.index.name must be date, df.columns must contain return, turnover, cost, bench.
return cost bench turnover date 2017-01-04 0.003421 0.000864 0.011693 0.576325 2017-01-05 0.000508 0.000447 0.000721 0.227882 2017-01-06 -0.003321 0.000212 -0.004322 0.102765 2017-01-09 0.006753 0.000212 0.006874 0.105864 2017-01-10 -0.000416 0.000440 -0.003350 0.208396
report_long_short_df –
df.index.name must be date, df.columns contain long, short, long_short.
long short long_short date 2017-01-04 -0.001360 0.001394 0.000034 2017-01-05 0.002456 0.000058 0.002514 2017-01-06 0.000120 0.002739 0.002859 2017-01-09 0.001436 0.001838 0.003273 2017-01-10 0.000824 -0.001944 -0.001120
show_notebook – Whether to display graphics in a notebook, default True. If True, show graph in notebook If False, return graph figure
- 返回:
图形结果
备注
- 一般图形
- std
- excess_return_without_cost
CAR`(累积异常收益)的 `标准差 无成本。
- excess_return_with_cost
CAR`(累积异常收益)的 `标准差 有成本。
- annualized_return
- excess_return_without_cost
CAR`(累积异常收益)的 `年化收益率 无成本。
- excess_return_with_cost
CAR`(累积异常收益)的 `年化收益率 有成本。
- information_ratio
- excess_return_without_cost
无成本的 信息比率(Information Ratio)。
- excess_return_with_cost
有成本的 信息比率(Information Ratio)。
想了解更多关于 信息比率(Information Ratio) 信息,请参考 Information Ratio – IR。
- max_drawdown
- excess_return_without_cost
CAR`(累积异常收益)的 `最大回撤 无成本。
- excess_return_with_cost
CAR`(累积异常收益)的 `最大回撤 有成本。
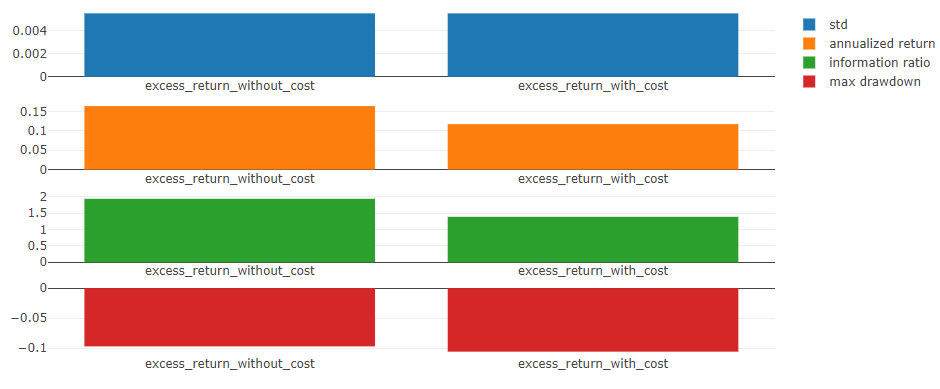
备注
- 年化收益/最大回撤/信息比率/标准差图表
X轴:按月分组的交易日
- Y轴:
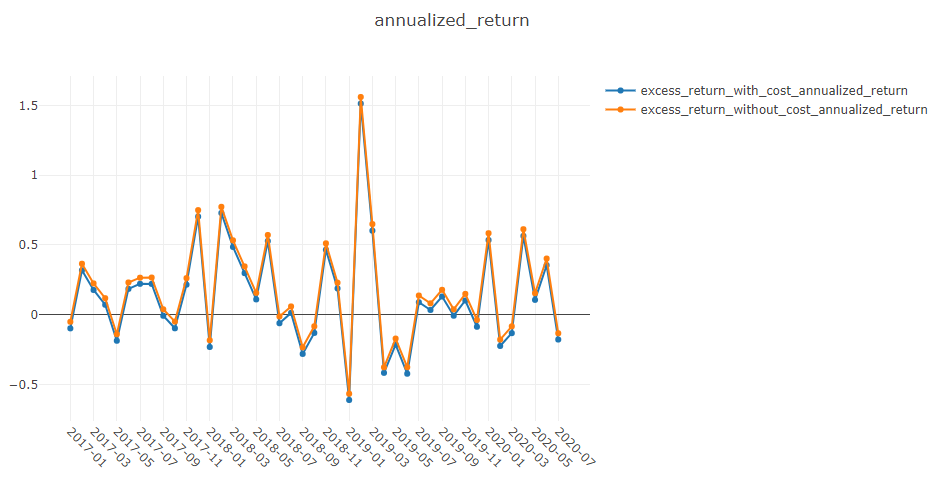
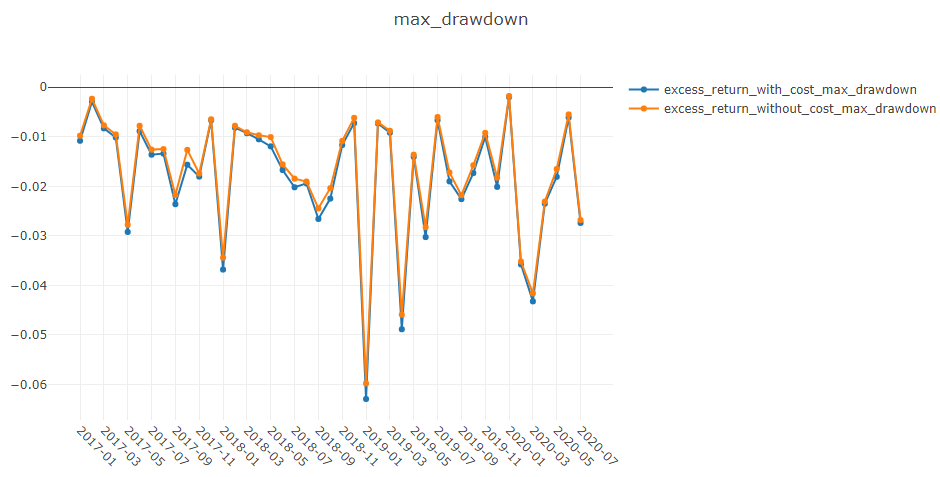
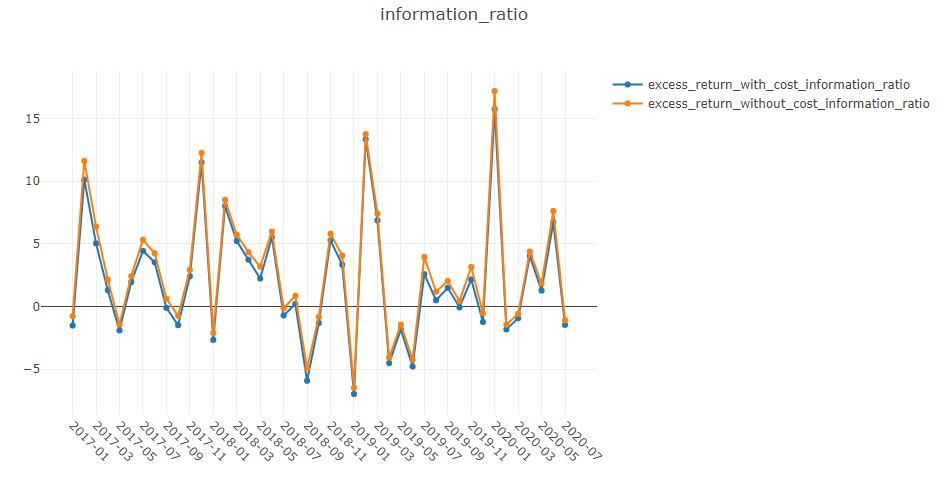
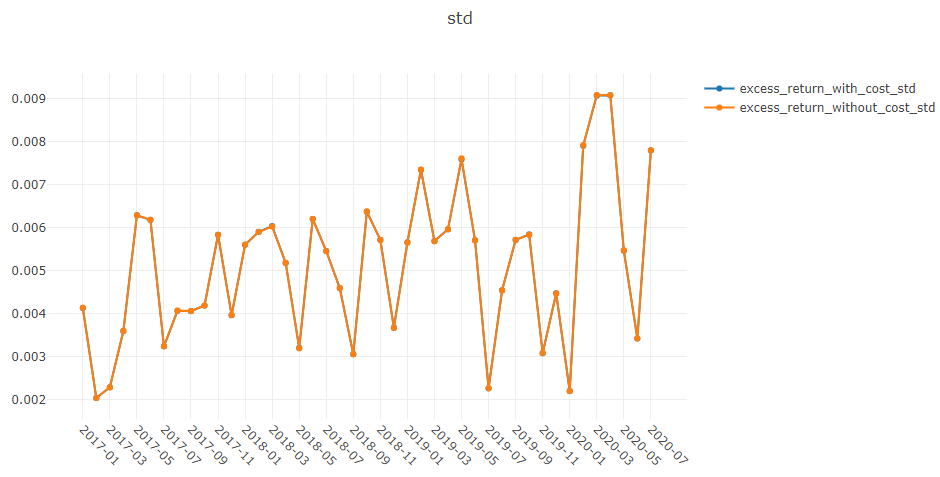
用法 analysis_model.analysis_model_performance
API
- qlib.contrib.report.analysis_model.analysis_model_performance.ic_figure(ic_df: DataFrame, show_nature_day=True, **kwargs) Figure
IC figure
- 参数:
ic_df – ic DataFrame
show_nature_day – whether to display the abscissa of non-trading day
**kwargs – contains some parameters to control plot style in plotly. Currently, supports - rangebreaks: https://plotly.com/python/time-series/#Hiding-Weekends-and-Holidays
- 返回:
plotly.graph_objs.Figure
- qlib.contrib.report.analysis_model.analysis_model_performance.model_performance_graph(pred_label: DataFrame, lag: int = 1, N: int = 5, reverse=False, rank=False, graph_names: list = ['group_return', 'pred_ic', 'pred_autocorr'], show_notebook: bool = True, show_nature_day: bool = False, **kwargs) [<class 'list'>, <class 'tuple'>]
Model performance
- 参数:
pred_label –
index is pd.MultiIndex, index name is [instrument, datetime]; columns names is [score, label]. It is usually same as the label of model training(e.g. “Ref($close, -2)/Ref($close, -1) - 1”).
instrument datetime score label SH600004 2017-12-11 -0.013502 -0.013502 2017-12-12 -0.072367 -0.072367 2017-12-13 -0.068605 -0.068605 2017-12-14 0.012440 0.012440 2017-12-15 -0.102778 -0.102778
lag – pred.groupby(level=’instrument’)[‘score’].shift(lag). It will be only used in the auto-correlation computing.
N – group number, default 5.
reverse – if True, pred[‘score’] *= -1.
rank – if True, calculate rank ic.
graph_names – graph names; default [‘cumulative_return’, ‘pred_ic’, ‘pred_autocorr’, ‘pred_turnover’].
show_notebook – whether to display graphics in notebook, the default is True.
show_nature_day – whether to display the abscissa of non-trading day.
**kwargs – contains some parameters to control plot style in plotly. Currently, supports - rangebreaks: https://plotly.com/python/time-series/#Hiding-Weekends-and-Holidays
- 返回:
if show_notebook is True, display in notebook; else return plotly.graph_objs.Figure list.
图形结果
备注
- 累计回报图形
- Group1:
排名比率 小于等于20%的股票组的 累计回报 系列
- Group2:
排名比率 在20%到40%之间的股票组的 累计回报 系列
- Group3:
排名比率 在40%到60%之间的股票组的 累计回报 系列
- Group4:
排名比率 在60%到80%之间的股票组的 累计回报 系列
- Group5:
排名比率 大于80%的股票组的 累计回报 系列
- long-short:
Group1 和 Group5 的 累计回报 之间的差异系列
- long-average:
Group1 和所有股票的平均 累计回报 之间的差异系列
- 排名比率 可以表示如下:
- \[ranking\ ratio = \frac{Ascending\ Ranking\ of\ label}{Number\ of\ Stocks\ in\ the\ Portfolio}\]
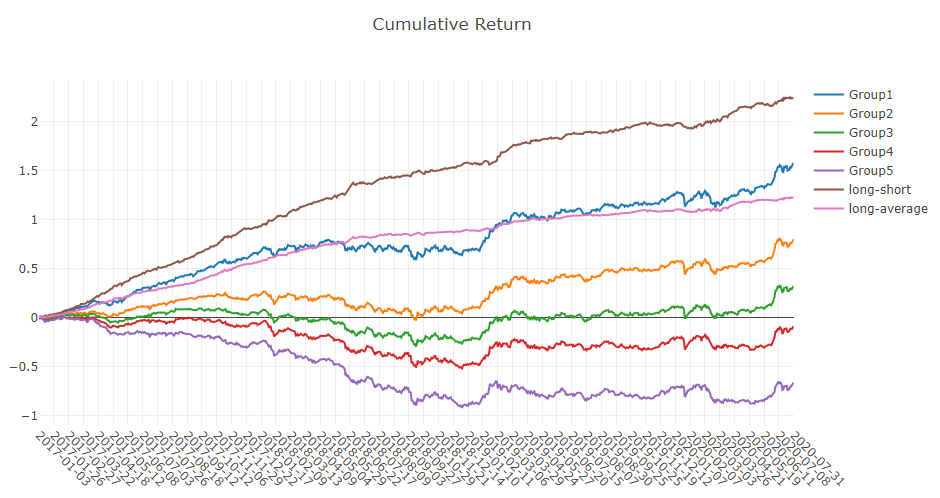
备注
- long-short/long-average
每个交易日的 做多-做空 / 做多-平均 回报分布
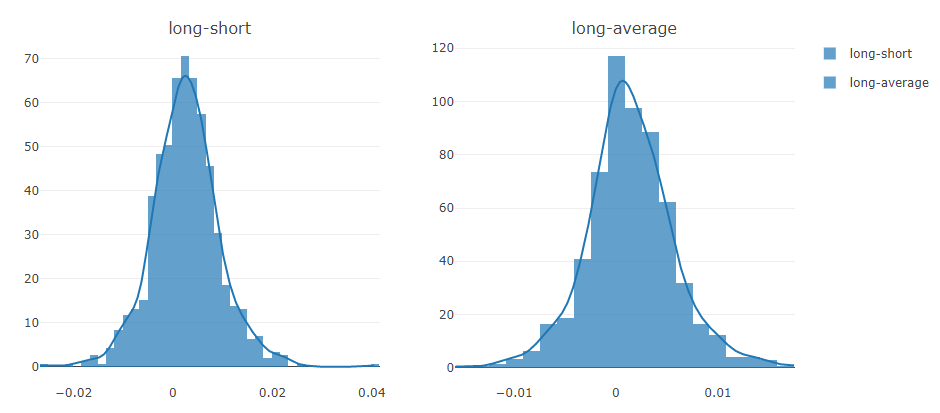
备注
- 信息系数
投资档案中股票的 标签 和 预测得分 之间的 Pearson相关系数 系列
这些图形可以用来评估 预测得分
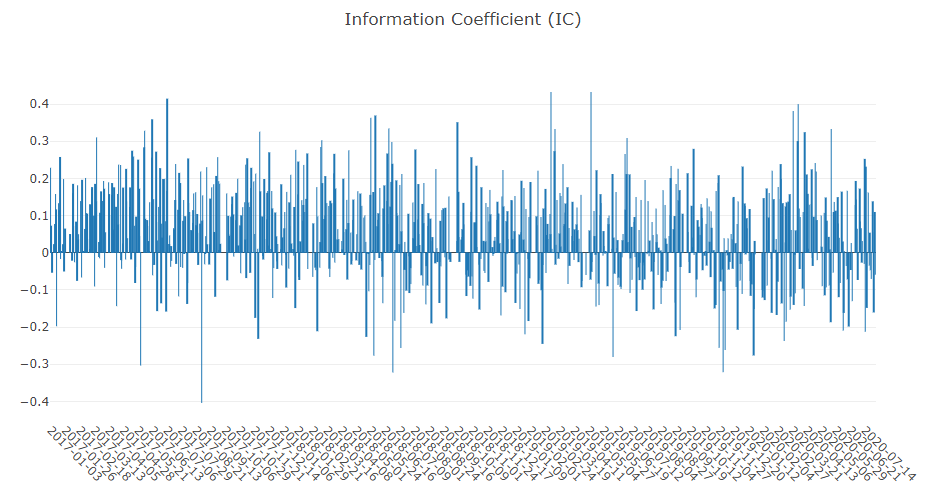
备注
- 月度IC
信息系数(IC)的月均值
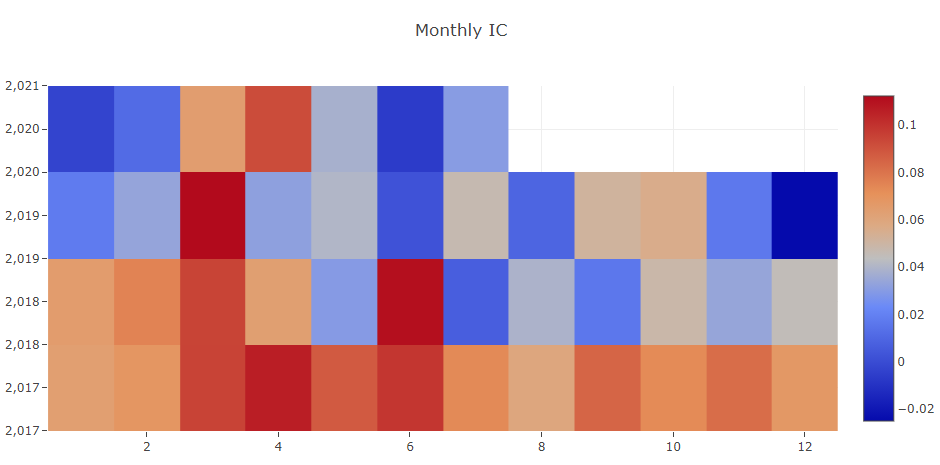
备注
- IC
每个交易日的信息系数(IC)分布。
- IC正态分布Q-Q图
用于描述每个交易日的信息系数(IC)的正态分布的量子-量子图。
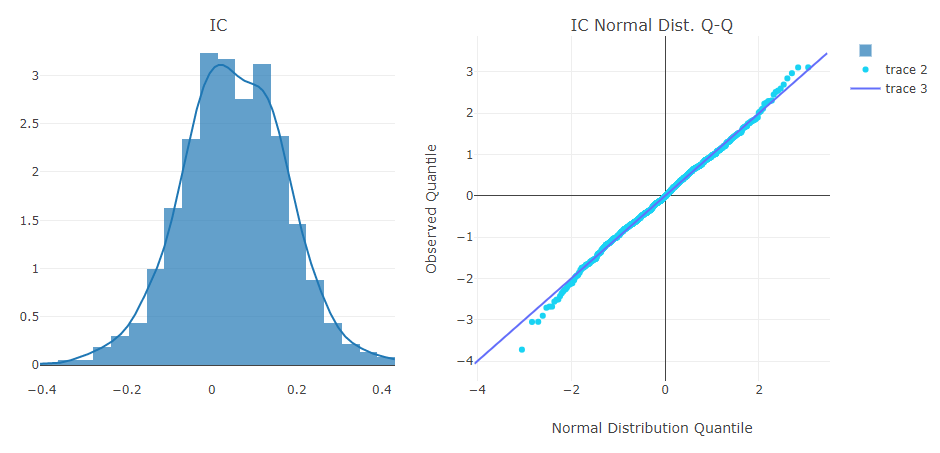
备注
- 自相关
每个交易日中股票组合的最新“预测得分”与“预测得分”在“滞后”时间天数前的“皮尔逊相关系数”系列。
可以使用图形报告来估计换手率。
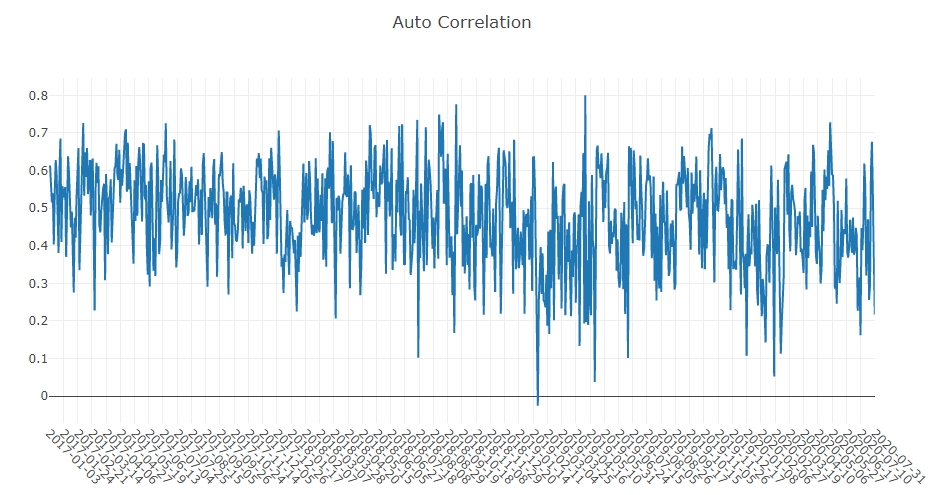